Kore.aiのNLPエンジンは、機械学習、ファンダメンタルミーニング、ナレッジグラフ(あれば)モデルを使用してインテントを一致させます。3つのKore.aiエンジンは最終的に完全一致または可能性のある一致のどちらかとしてKore.aiランキングおよび解決コンポーネントに結果を提供します。ランキングおよび解決はNLP計算全体の最終的な結果を決定します。
動作
NLPエンジンは、機械学習、ファンダメンタルミーニング、ナレッジグラフ(Botに含まれる場合)モデルを使用したハイブリッドアプローチによって、関連性に関する一致するインテントをスコア化します。モデルは、ユーザーの発話を可能性のある一致または完全一致のいずれかに分類します。 完全一致は、高い信頼度スコアを取得し、ユーザーの発話に完全に一致すると見なされます。公開済みのBotでは、ユーザー入力が単一の完全一致と一致する場合、Botは直接タスクを実行します。発話が複数の完全一致と一致する場合、エンドユーザーが選択できるようにオプションとして送信されます。 一方、可能性のある一致とは、ユーザー入力に対してある程度スコアが高いインテントを指しますが、完全一致と呼ぶには十分でないインテントのことです。内部的には、システムは、スコアに基づいて、可能な一致をさらに通常の一致と不正確な一致に分類します。公開済みのBotでエンドユーザーの発話が可能性のある一致を生成していた場合、Botはこれらの一致をエンドユーザーに「Did you mean?」として送信します。 ランキングおよび解決に基づいて、エンジン間の上位インテントを確認します。プラットフォームがあいまいさを検出した場合、あいまいさのダイアログが開始されます。プラットフォームは、ユーザーの発話に対する単一の上位インテントを確認できない場合、これら2つのシステムダイアログのいずれかを開始します。
- あいまい性解消ダイアログ:エンジン間で返された完全一致が複数ある場合に開始されます。このシナリオでは、Botは実行する完全一致を選択するようユーザーに求めます。NLP標準応答から、ユーザーに表示されるメッセージをカスタマイズすることができます。
- 「Did You Mean」ダイアログ:ランキングおよび解決が複数の上位インテントを返した場合、あるいは唯一の上位インテントが、KGエンジンのスコアがしきい値の下限と上限の間にあるFAQである場合に開始されます。このダイアログは、完全であるか不明なインテントと一致するものを検出したことをBotがユーザーに知らせるものであり、ユーザーに先へ進んための選択を促します。このシナリオでは、開発者はこれらの発話を識別し、Botをさらにトレーニングする必要があります。ユーザーに表示されるメッセージは、NLPの標準応答からカスタマイズすることができます。
しきい値および設定
ランキングおよび解決エンジンは以下の手順で設定することができます。
- しきい値を設定するBotを開きます。
- サイドナビゲーションパネルにカーソルを合わせ、自然言語 > トレーニングをクリックします。
- しきい値および設定タブをクリックします。
- ランキングおよび解決エンジンセクションでしきい値を設定することができます。
- 可能性のある一致の近似度は、スコアの高い上位インテントとその次の可能性のあるインテントを同じように重要なものとみなすために許容される最大差を定義します。プラットフォームのバージョン7.3以前では、この設定はファンダメンタルミーニングセクションで行うことができました。
- 依存構造解析モデルは、ファンダメンタルミーニングモデルによるインテント認識と同様に、ランキングおよび解決エンジンによるインテントの再スコアリングを有効にするためのものです。この設定はデフォルトでは無効になっており、設定を行う必要があります。詳細は以下を参照してください。
依存構造解析モデル
このプラットフォームには、ファンダメンタルミーニングエンジンとランキングおよび解決エンジンによる、2つのインテントのスコアリングモデルがあります。
- 最初のモデルは、主に単語の存在、発話の中での単語の位置などに依存してインテントを判断し、ファンダメンタルミーニングエンジンのみによってスコア化されます。こちらがデフォルト設定になっています。
- 2つ目のモデルは、依存マトリックスに基づいており、インテントの検出は、単語やその相対的な位置、そして最も重要とされる、文中のキーワード間の依存関係に基づいて行われます。このモデルでは、インテントはファンダメンタルミーニングエンジンによってスコアリングされ、その後、ランキングおよび解決エンジンによって再ドスコアリングされます。
依存構造解析モデルは、自然言語 > トレーニング > しきい値および設定のランキングおよび解決セクションから有効化や設定を行うことができます。 注:この機能はプラットフォームのバージョン7.3で導入され、一部の言語でのみサポートされています。対応言語についてはこちらを参照してください。 依存構造解析モデルは、以下のように設定することができます。
NLP検出
自然言語分析の結果、以下のようなシナリオになります。
- FM、ML、またはKGエンジンで完全一致を識別するNLP分析
- 可能性のある一致を返し、単一の一致を選択する、複数のエンジンを用いたNLP分析
- 可能性のある一致を返す複数のエンジンと複数の結果を返す解決を用いたNLP分析
- 一致しないNLP分析
ここでは、上記のそれぞれのケースについて説明します。 NLP検出を理解するために、以下の詳細を含む銀行Botを例として見てみましょう。
シナリオ3 – 完全一致を識別するKG 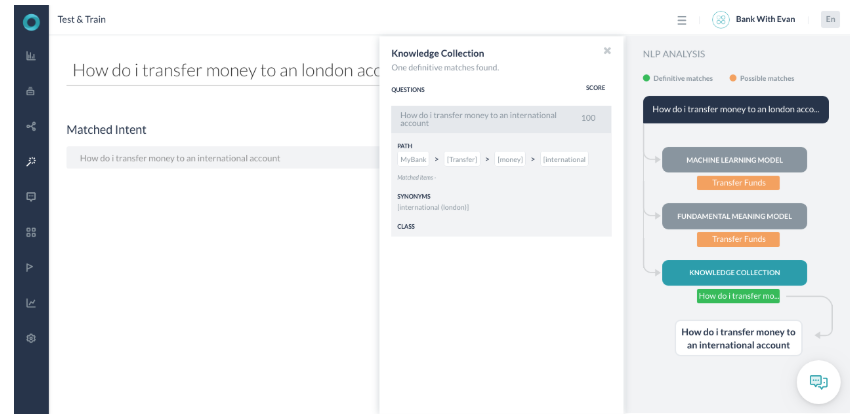
- ユーザーの発話は「How do I make transfer money to a London account?」です。
- ユーザーの発話には、このナレッジグラフのインテントパスである「Transfer」、「Money」、「International」に一致するために必要なすべての用語が含まれています。
- 「international」という用語は、ユーザーが発話の中で使用した「London」の同義語として識別されます。
- 100%のパス用語が一致したため、パスが修飾されました。信頼度スコアリングの一部として、ユーザークエリの用語は実際のナレッジグラフの質問の用語と似ており、そのため100のスコアが返されます。
- 返されたスコアが100以上の場合、インテントは完全一致とマークされ、選択されます。
- FMエンジンは、キー用語であるTransferがユーザーの発話の中に存在するため、可能性のある一致と判断しました。
- MLエンジンは、発話がトレーニングされた発話と完全に一致しなかったため、可能性のある一致と判断しました。
シナリオ4 – 可能性のある一致を返す複数のエンジン 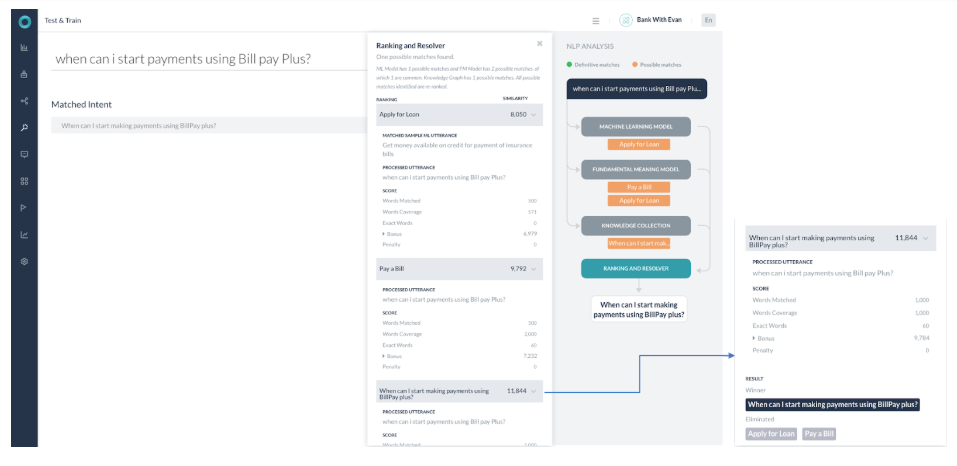
- 3つのエンジンはすべて可能性のある一致を返し、完全一致を返しませんでした。。
- MLモデルには可能性のある一致が1件あり、FMモデルには可能性のある一致が2件あり、そのうち1件は共通しています。ナレッジグラフには可能性のある一致が1件あります。識別された可能性のある一致はすべて、ランキングおよび解決で再ドランク付けされます。
- ランキングおよび解決コンポーネントは、ナレッジグラフエンジンから単一の一致(タスク名 – 「When can I start making payments using BillPay plus?」)の最高スコアを返しました。他の可能性のある一致のスコアは、上位スコアの2パーセンタイルよりも低いため、無視されます。この場合、上位は「KG」に返されたクエリであり、ユーザーに提示されます。
- ユーザーの発話のほとんどのキーワードはKGクエリのキーワードをマッピングしますが、これらは完全一致ではありません。理由は以下のとおりです。
- パス用語の一致数は100%ではありません。
- KGエンジンは64.72%の可能性を示すスコアを返しました。「bill pay」の代わりに「Billpay」という単語を使用していた場合、スコアは87.71%になっていたはずです。(それでも100%ではありません)
- スコアが60%~80%の場合、クエリは「Did-you-mean」ダイアログの一部として表示され、完全な一致として表示されません。スコアが80%を超えていた場合、プラットフォームは「Did-you-mean」ダイアログを使用して再度確認することなく回答を表示していたはずです。